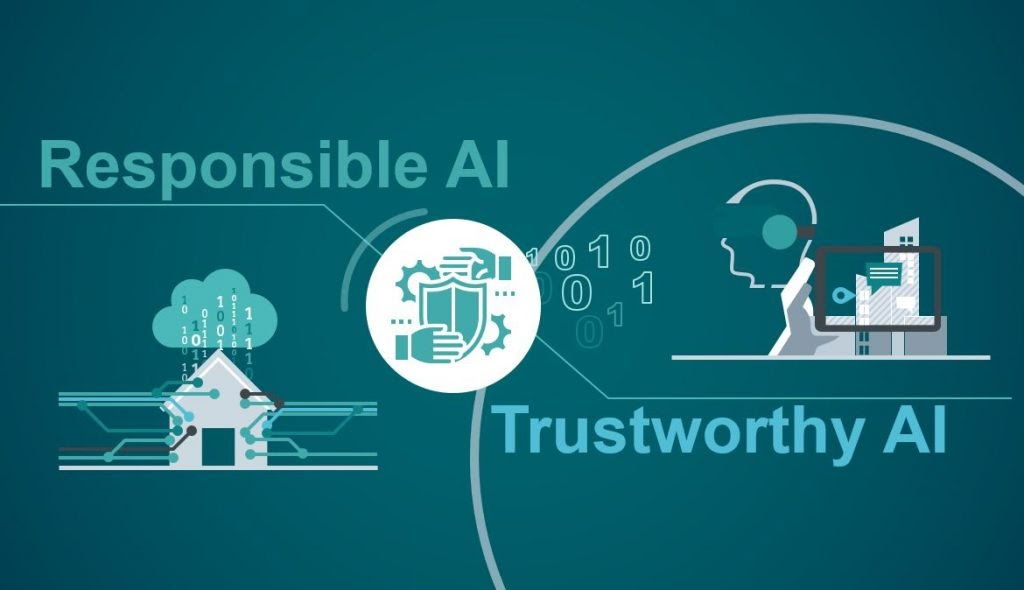
Responsible AI is not a far-off idea; it’s present, changing choices in health care, money matters, learning, and other fields. But as AI tools get stronger, the duty to make sure they are used rightly grows too. This is where Fair AI steps up, a thought aimed at making and using AI tools that are just, clear, and good for everyone.
Table of Contents
What is Responsible AI?
Careful AI means making AI systems that are safe, honest, and in line with human beliefs. It makes sure that AI doesn’t bring about unplanned harm, honors privacy, helps fairness, and is responsible to people. Instead of just looking at what AI can make, Responsible AI points out what AI must do, putting people, fairness, and lasting effects first before quickness or money.
Why Responsible AI Matters
As AI gets more stuck in society, from hiring tools to face checks and money scores, its moral effects are large. Unfair programs, no clear view, and wrong use of data can cause unfairness, loss of trust, and even law issues. Smart AI helps lessen these dangers, making sure tech works for people, not the other way.
Core Principles of Responsible AI
1. Fairness
AI tools should treat all people the same. This means not having unfair views based on color, sex, age, or other protected traits. Builders must often look at and fix unfair training in͏fo and way of doing things.
2. Transparency
Folks need to grasp how AI picks. Good AI highlights simple talk, easy-to-understand rules, and the chance to ask or challenge choices made by AI machines.
3. Accountability
Groups are in charge of their AI tools. This means giving roles for watching over things, writing down choices made during growth, and being ready to deal with any bad results.
4. Privacy and Security
AI should honor user info by following strict privacy rules and making sure data is safe. Things like hiding identities and asking for agreement to use data are key parts of careful design.
5. Reliability and Safety
AI must work as planned in different situations. Hard testing, ongoing watching, and added safety nets help make sure systems don’t act strangely or hurt users.
Challenges in Practice
Even if the aims of Good AI are clear, putting them into action can be hard. Main issues include:
- Opaque models (like deep learning black boxes)
- Bias in training data
- Lack of diverse development teams
- Quick speed of AI changes, but slower rules making
Getting past these bumps needs a team effort, bringing in data workers, moral thinkers, rule makers, and users.
Real-World Examples
- Microsoft mixes fair check methods into its AI making process and uses tools like “Fairlearn” to find unfairness in algorithms.
- Google started its PAIR plan to make AI tools that are friendly and clear.
- IBM gives clear info with “AI FactSheets,” that tell how their models function and what data they use.
These plans show that with good setups, big groups can be led by Monday in making caring tech.
Shaping a Future with Ethical AI
Ethical AI isn’t a one-time project; it’s a continuous commitment. As AI becomes more independent, the ethical dilemmas we face will only become more complex. In the future, we can expect:
- Universal AI standards and regulations
- Enhanced industry-wide cooperation
- AI ethics training as a common practice
Ultimately, beneficial AI hinges on trust. When people can rely on AI with confidence, technology becomes a tool for collective progress rather than a source of peril.
Conclusion
AI is changing our world, but how it͏ changes it is still up to us. Responsible AI gives a way forward, making sure that as machines become cleverer, our society gets smarter. By putting right and wrong into design, honesty into systems, and responsibility into actions, we make a future where AI helps everyone