"Demand for Generative AI in Enterprises"
Snowflake, a leading cloud data platform, unveils new updates to meet the growing demand for generative AI solutions in enterprises. Discover how Snowflake’s advancements are empowering businesses to harness the power of AI and unlock innovative possibilities.
Snowpark Container Services have emerged as a game-changer for enterprises dealing with complex language models. These container services provide a scalable and efficient infrastructure to deploy and manage large language models. With Snowpark, organizations can harness the full potential of these models without the need for extensive hardware resources or infrastructure setup. This breakthrough technology opens up new possibilities for enterprises to leverage AI and drive innovation in various domains.
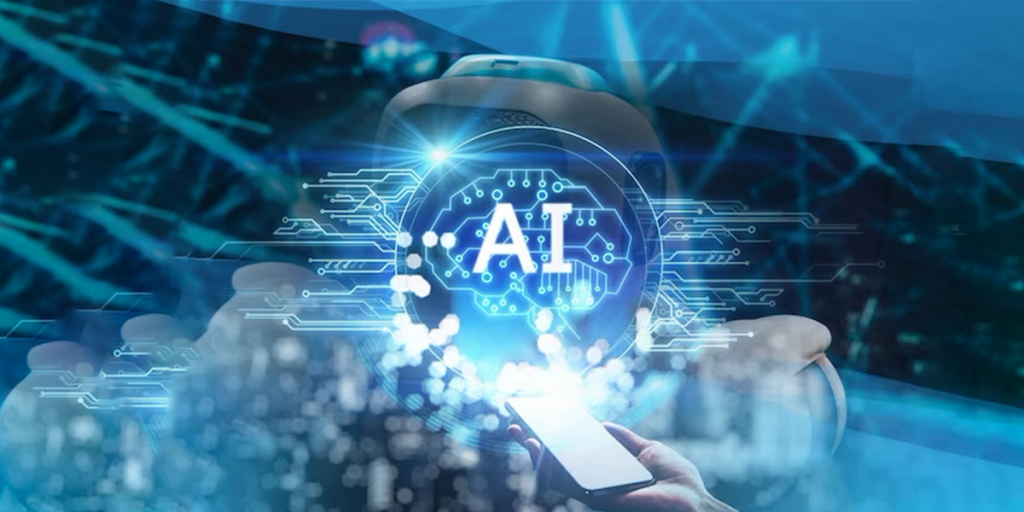
this topic created by infoworld.
In today’s rapidly evolving digital landscape, enterprises are increasingly turning to generative AI to fuel innovation, gain a competitive edge, and uncover new business opportunities. Recognising this demand, Snowflake, the renowned cloud data platform, has introduced significant updates to address the growing need for generative AI solutions. This article delves into the latest developments by Snowflake and their implications for businesses embracing the power of artificial intelligence.
Snowflake’s enhanced capabilities cater to the unique requirements of enterprises seeking to leverage generative AI technologies. With these updates, Snowflake offers a scalable and secure environment for businesses to develop and deploy AI models that drive automation, creativity, and efficiency across various domains. By leveraging Snowflake’s advanced data management infrastructure, enterprises can effectively harness the potential of generative AI to transform their operations.
The updates introduced by Snowflake empower businesses to tackle complex challenges by providing a streamlined approach to data ingestion, storage, processing, and analysis. With the ability to handle massive datasets, Snowflake allows enterprises to derive valuable insights and train sophisticated generative AI models. This enables businesses to create personalized customer experiences, optimize resource allocation, enhance product design, and revolutionize decision-making processes.
Snowflake’s updates also focus on enhancing collaboration and accessibility within the generative AI ecosystem. By providing seamless integration with popular AI frameworks and tools, Snowflake facilitates efficient model training, testing, and deployment. This enables data scientists and AI practitioners to leverage their preferred frameworks while benefiting from Snowflake’s robust infrastructure and data management capabilities.
Furthermore, Snowflake’s advanced security features ensure the protection and privacy of sensitive data used in generative AI applications. With comprehensive data governance controls, encryption, and compliance measures, Snowflake enables enterprises to adhere to stringent security standards and regulatory requirements. This instills trust and confidence among businesses seeking to leverage generative AI while safeguarding their valuable data assets.
In conclusion, Snowflake’s updates geared towards meeting the rising demand for generative AI in enterprises demonstrate their commitment to empowering businesses with cutting-edge AI capabilities. By leveraging Snowflake’s cloud data platform, businesses can unlock the full potential of generative AI to drive innovation, enhance operational efficiency, and gain a competitive advantage in today’s data-driven world. With Snowflake’s robust infrastructure, seamless integration, and advanced security features, enterprises can embark on their generative AI journey with confidence, propelling them towards a future of limitless possibilities.
"Meeting the Rising Demand for Generative AI in Enterprises"
this video created by CNBC Television.
"Nvidia Partnership: Revolutionizing LLM Training with Advanced Technology"
Nvidia, a global leader in AI computing technology, has formed a groundbreaking partnership aimed at transforming the landscape of Legal Language Modeling (LLM) training. This collaboration brings together Nvidia’s cutting-edge technology and the expertise of legal professionals to revolutionize the way legal language models are developed and utilized. With this innovative partnership, the legal industry can expect accelerated advancements in LLM training, paving the way for more efficient and accurate legal language processing.
"Unleashing the Power of Unstructured Data: Document AI Generates Actionable Insights"
In today’s data-driven world, a significant portion of valuable information is hidden within unstructured data such as documents, contracts, and reports. Extracting meaningful insights from this data has traditionally been a time-consuming and resource-intensive task. However, with the advent of Document AI, businesses can now harness the power of advanced machine learning techniques to unlock actionable insights from unstructured data. Document AI empowers organizations to streamline processes, make informed decisions, and drive innovation by transforming unstructured data into structured, actionable information.
"MLOps Tools and Updates: Streamlining Machine Learning Operations"
MLOps (Machine Learning Operations) has become a critical aspect of managing and scaling machine learning projects. To address the evolving needs of the AI community, several innovative MLOps tools and updates have emerged, revolutionizing the way organizations deploy and manage machine learning models. This article explores the latest advancements in MLOps and highlights how these tools streamline machine learning operations for improved efficiency and productivity.
As the field of machine learning continues to advance, organizations are faced with the challenge of efficiently deploying and managing their models in real-world scenarios. MLOps tools bridge the gap between data science and production, providing a comprehensive set of practices and technologies to streamline the entire machine learning lifecycle. From data preparation and model training to deployment and monitoring, MLOps tools automate and simplify key tasks, enabling organizations to accelerate their AI initiatives.
One notable update in the MLOps landscape is the emergence of powerful model deployment and serving platforms. These platforms offer seamless integration with popular machine learning frameworks and provide robust infrastructure to host and serve models at scale. With features like automated scaling, versioning, and containerization, organizations can easily deploy and manage their models in production environments, ensuring reliable and efficient inference.
Another significant advancement in MLOps is the integration of monitoring and observability capabilities. Real-time monitoring allows organizations to track model performance, detect anomalies, and ensure models are functioning optimally. By leveraging metrics, logs, and alerts, MLOps tools provide valuable insights into model behavior, facilitating proactive maintenance and enabling organizations to make data-driven decisions.
Additionally, MLOps tools have evolved to address the challenges of reproducibility and version control. These tools provide robust mechanisms for managing data and model artifacts, enabling organizations to track changes, reproduce experiments, and collaborate effectively. By ensuring consistency and reproducibility, organizations can maintain transparency and trust in their machine learning workflows.
With the increasing complexity of machine learning projects, organizations are also turning to automated testing and continuous integration/continuous deployment (CI/CD) pipelines. MLOps tools integrate seamlessly with CI/CD frameworks, allowing organizations to automate testing, code review, and model validation processes. This ensures the smooth and efficient deployment of models, reducing the risk of errors and improving overall software quality.
In conclusion, the rapid evolution of MLOps tools and updates is revolutionizing the way organizations manage and operationalize machine learning projects. From model deployment and serving platforms to monitoring, observability, and automation, these tools empower organizations to streamline their machine learning operations and drive innovation at scale. By leveraging the latest advancements in MLOps, organizations can accelerate their AI initiatives, improve productivity, and deliver impactful machine learning solutions in a reliable and efficient manner.